Machines don't understand being wet
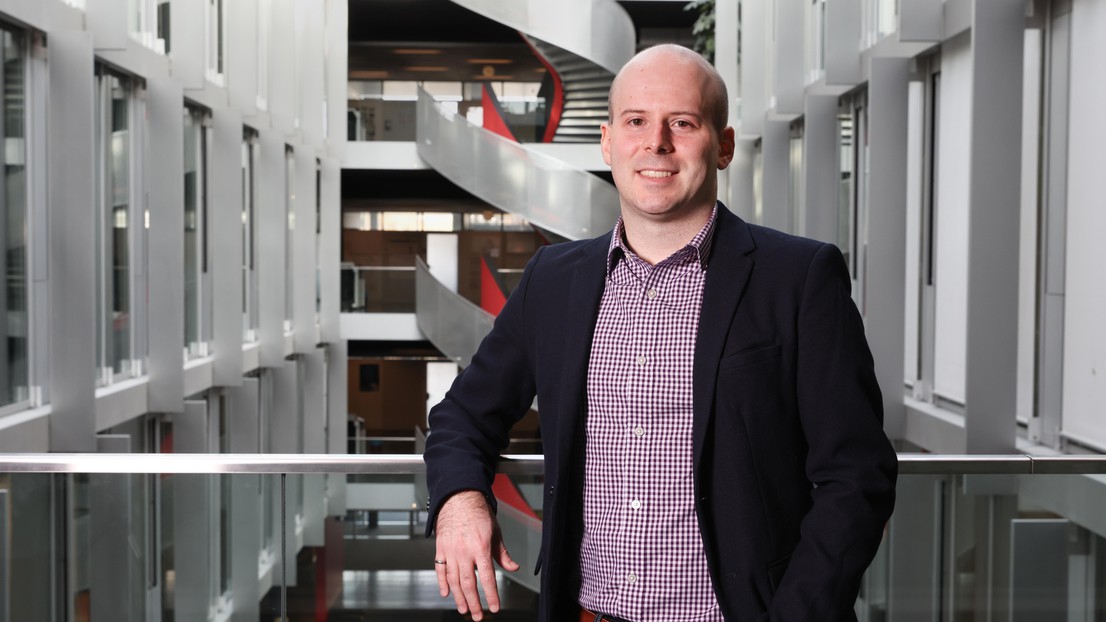
Assistant Professor Antoine Bosselut © Alain Herzog / EPFL 2021
Assistant Professor Antoine Bosselut, who joined EPFL in September, was recently named one of Forbes Magazine’s prestigious ‘30 under 30 Europe in Science and Healthcare’ for his research in Natural Language Processing on whether AI systems learn common sense from language?
Eighteen months ago Antoine Bosselut accepted a job at EPFL in the School of Computer and Communication Sciences (IC) to lead the new Natural Language Processing (NLP) group, a move from Stanford University in California delayed by the Covid-19 pandemic. Finally on campus, he says it’s a pleasure to be working beyond the four walls of his apartment, and incredibly exciting to be building an NLP-focused environment at the university.
“The simplest way NLP can be described is how do we get machines to understand human language and then use that to understand us or communicate with us, such as in conversational systems like Alexa or Siri or tools like Google Translate. It's really the study of any problem that involves understanding human language to augment human capabilities or provide insights into the way that humans think and feel.”
Bosselut’s research currently focuses on what is outside explicit language, that is, the background knowledge that underlines the things we tend to say, and then how to give machines that same set of assumptions, pre- and post-conditions, that same understanding of the world around them in order to be able to more clearly understand the things that humans tell them and produce language that makes sense.
“If I give a simple story of ‘oh, it's going to snow tonight, I'm going to have to wake up half an hour earlier tomorrow’, you can immediately create different narratives as to why that makes sense. You might have to shovel your car out of the snow; if you take public transport it might be a bit slower; there could be ice on the sidewalks so you're going to have to be more careful. You are aware of all these possible conditions that will exist based on the first statement that makes the second one sensible,” Bosselut says.
But machines don’t yet have those innate human assumptions. “A good analogy is that they might know that when it's raining water falls from the sky, and separately that people get wet but they won’t learn that people are getting wet because water is falling from the sky. My research tries to fill the gaps in that narrative or find methods that allow machines to learn how to fill in the gaps to pick up these connections in the first place,” he continues.
“We've made tremendous progress in this area over the last few years. One of the things that we've been able to show in our own research is that the more text that these large-scale NLP systems read, the more they are able to learn this background information. If you read 1000 times about it raining outside and each time a bit more information is given - drops were falling, it was pouring, I put on my raincoat, I opened my umbrella - it allows the machines to gain a much broader understanding of the types of situations that they’re reading about.”
Despite this advance in understanding, Natural Language Processing as a field has only recently become mainstream very quickly, and Bosselut believes that in the coming decade there will be considerable exploration into some of the negative capabilities that it has unleashed, often caused by a misunderstanding of how algorithms make the predictions they do and how they connect the dots.
“In some instances, the capabilities of these systems have outrun our understanding of them. For example, nobody knows how the GPT-3 model, a very large NLP system, functions internally. It’s behind a firewall for IP reasons, so people can’t study what it does, but it’s being used to drive future products. Should these products be shared with other people if we don’t understand the model supporting them?” Bosselut asks.
“There are also dangers,” he continues, “because some people are driven by some of the worst incentives out there. My hope is that, at the very least, if we can understand how these machines understand things, we can design more and better regulation to reduce the worst that humans can bring out of them.”
“I think climate change is a perfect analogy because the playbook around some of the counter narratives of AI dangers is similar - ‘we don't understand how it happens so why make changes to mitigate something that we don't understand?’ I feel that maybe in 10 years we'll look back and think that a degree of ignorance, intentional or not, was allowed to give plausible deniability for some of the worst things that these technologies can cause,” he poses.
Recently for his work Bosselut joined the ranks of Forbes Magazine’s prestigious ‘30 under 30 Europe in Science and Healthcare’, recognizing him as one of the brightest and most influential researchers on the continent. It’s great kudos for the research and thought leadership Bosselut was already undertaking but he is already looking forward, “As much as I can imagine the opportunities this award will create I would like to think that it’s an effect as opposed to a cause and it’s really a validation of the research that I, and the fantastic people I work with, are trying to do here.”