Paper on Dynamic Credit Collections Accepted at Management Science
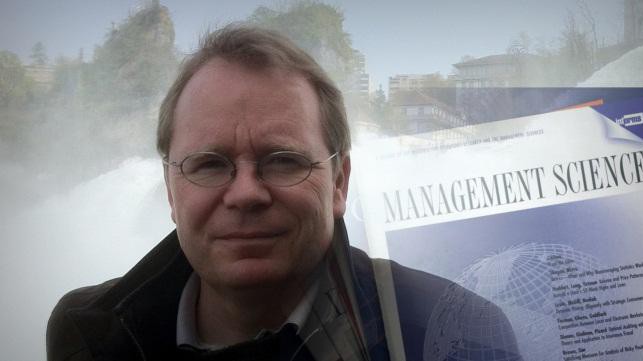
Prof. Thomas Weber © 2015 EPFL
Recent research by Prof. Thomas Weber and his former student, Prof. Naveed Chehrazi (UT Austin), on the “Dynamic Valuation of Delinquent Credit-Card Accounts” has been accepted for publication by Management Science, the leading INFORMS journal. In their paper, the authors introduce a dynamic collectability score (DCS), which can be used to evaluate the probability that a delinquent credit-card account repays an outstanding balance. The parameters of the underlying model, based on stochastic point processes, can be estimated using techniques provided by the authors. The theoretical results are applied to a large data set obtained from a major credit-card issuer.
In the United States alone, each year more than $15 billion of overdue credit-card debt is placed in collections and only a fraction of that amount is ever recovered, usually with the help of professional credit-collection agencies. The standard procedure for recovering the outstanding funds is that after a certain time past the due date (e.g., 60 days) the bank would declare an account “delinquent” and place it in “collections.” At that point, professional collectors—within or, more commonly, outside the issuing bank—would try to establish contact with the account holder and, if successful, attempt to determine a plan for a full repayment of the overdue balance.
The Scoring Problem. To determine how much effort to spend, at any point in time, on the collection effort for a given delinquent account, it is of key importance for a bank to have reliable estimates about the likelihood with which accounts pay back a given fraction of the outstanding balance over a given time horizon. Estimates should be specific for an account and use all available information. One of the challenges is that information typically comes from very different sources. First, there are the repayment history of the account under consideration and the repayment histories of similar accounts. Second, there are pieces of account-specific information, such as the demographics of the account holder (e.g., age and marital status) or the number of credit lines, the credit rating (FICO score) and the status of the holder’s mortgage, which may all be indicative for the collectability of the account. Third, there is the collection strategy
of the agency or bank, which may include phone calls and an escalating series of letters, at times culminating in legal action. Finally, there are dynamic macroeconomic variables, such as the interest rate or indices for consumer prices and stockmarket performance, which are correlated with the repayment behavior. The scoring problem in credit collections amounts to the question of how, at any point in time, to integrate all the available information into a reliable estimate of the collectability of a given account or a given group of accounts, as defined by the probability that a certain fraction of the outstanding balance will be paid back over a certain horizon. Chehrazi and Weber provide an answer to this problem by constructing a Dynamic Collectability Score (DCS).
Why is the scoring problem difficult? Current bank-internal scoring (BIS) systems are surprisingly poor in predicting repayment behavior, given the amounts that are at stake. Until about a decade ago, banks with billions of dollars in outstanding debt were not even using basic (“Bayesian”) probability updates to change their repayment estimates over time. In other words, banks failed to update their collectability estimates despite the availability of a rich stream of dynamic information, including the account’s behavior, experience derived from similar accounts, and the dynamic covariates. The DCS scoring methodology by Chehrazi and Weber provides a significant improvement over bank-internal scoring methods, as evidenced by substantial lifts in the so-called ROC curves, which measure the true positives vs. the false positives when predicting which account will repay a balance or not.
How does the DCS work? Using a self-exciting point process, governed by a stochastic differential equation, the authors model the fact that the likelihood (or “stochastic intensity”) of repayments increases after an account holder starts
paying back. The repayment probability is also increased by collection actions. Dynamic covariates evolve separately and determine the long-run intensity of the repayment process. Using the available time-series data, Chehrazi and Weber provide several methods to statistically identify the parameters of the stochastic model. The DCS score is then obtained by calculating the probability of repayments. Because of the generality and flexibility of the DCS as a repayment measure, it also allows for a dynamic valuation of overdue credit-card accounts.
Implications. The DCS improves many aspects of collection management; it can be viewed as a fundamental tool for optimizing the collections process. For example, the DCS can be used to optimize the commission rates paid to credit-card agencies or to find, based on the dynamic account valuation, a settlement rate at which the bank would rationally want to accept partial repayment instead of waiting for the outcome of a protracted collections process (for an earlier, static but robust treatment of the settlement problem, see Chehrazi and Weber 2010). By providing estimates for the banks payoffs after an account enters default, the DCS also informs the underwriting process. Lastly, because the entire methodology is conditioned on the sequence of account-treatment actions the bank plans to follow, it is possible to optimize the treatment process so as to maximize the expected return (also known as the “spin” in the industry) from any given account. The latter is the subject of follow-on research by authors in collaboration with Peter Glynn (Stanford University).
References
- Chehrazi, N., Weber, T.A. (2015) “Dynamic Valuation of Delinquent Credit-Card Accounts,” Management Science, Forthcoming. [For a preprint see: http://dx.doi.org/10.2139/ssrn.1868581 ]
- Chehrazi, N., Weber, T.A. (2010) “Monotone Approximation of Decision Problems,“ Operations Research, Vol. 58, No. 4, Part 2 of 2, pp. 1158—1177.